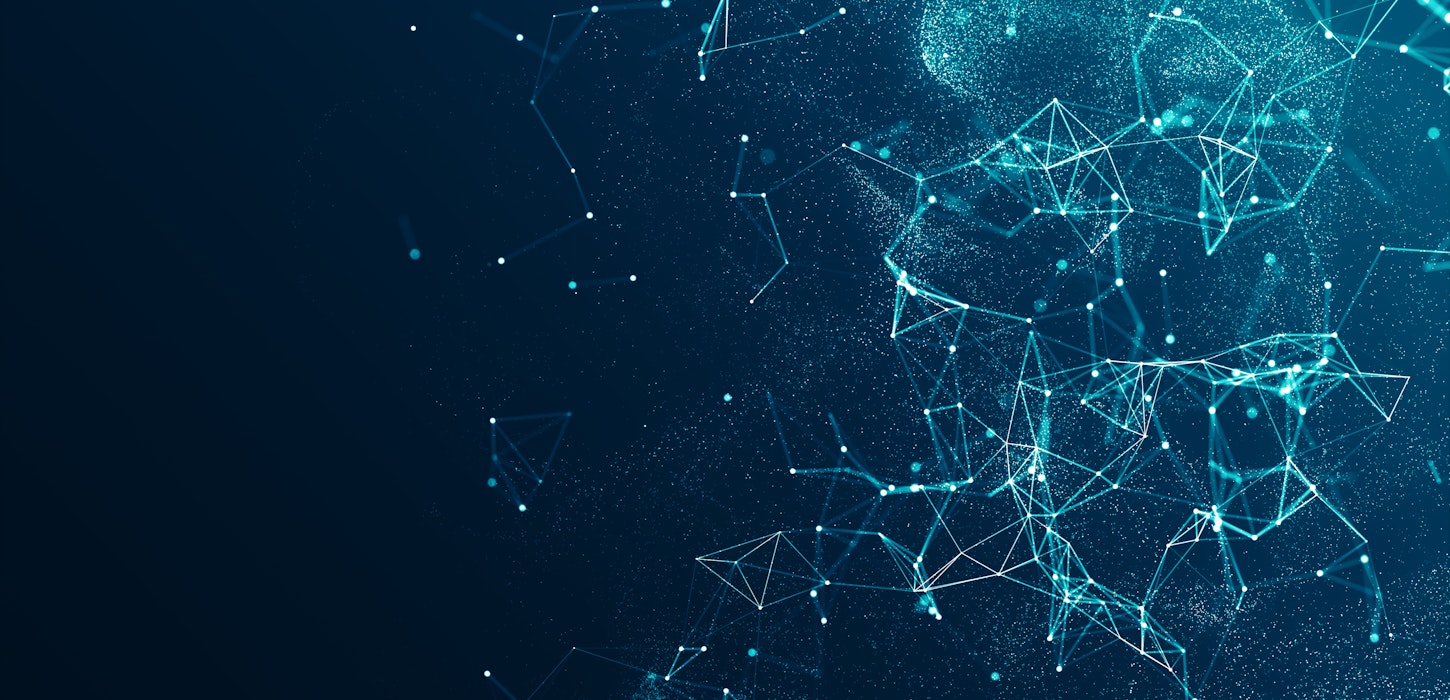
AI solutions: To build or to buy? That is the question
AI promises transformative capabilities for organisations working in the risk, security, and intelligence domains, but its integration into frameworks and processes requires careful consideration if it is to be achieved successfully. One of the most important things that leaders contend with when considering AI solutions is the ‘build vs buy’ conundrum, writes Seb Matthews, Adarga’s Vice President of Mission Engineering.
AI’s ability to process data at an eye-watering scale can be a significant force multiplier alone, and its ability to recognise patterns, extract entities, infer, and make predictions – among many other attributes – means that the technology is a genuinely useful ‘augmentee’ to analysts, enabling them to work at a scale and speed not practically possible with traditional methods and human endeavour alone, and to uncover insights that would otherwise remain buried.
Successfully bringing AI to life within organisations requires a nuanced understanding of the challenges in doing so, nowhere is this more important than when considering whether to build or buy capability. Both approaches can come with drawbacks and compromises, and there are several factors that determine which direction of travel is most suitable.
The first of which is determining the desired outcome – what is it that an organisation is trying to achieve? If there is a solution that meets your requirements off-the-shelf then it is unwise to try and create that capability in-house or modify another to replicate it – the reward is unlikely to be worth the risk and cost. The challenge for organisations arises when their needs cannot effectively be addressed by generic AI tooling alone. For example, there may be a very specific capability or heavy reliance on the domain knowledge of individuals that must be considered.
Heavily tailored solutions to meet specific challenges are of course feasible, but picking the right technology provider to do this is key. If approached wrongly you could end up with off-the-shelf software that has been ‘bent’ into shape for specific needs, which makes it very hard to ‘bend’ it into a new shape if objectives change. Of course, software by its very nature is iterative and those tech providers built to deploy rapid innovation and iterate at speed can make this approach a very valuable and flexible endeavour.
Understanding the contextual and the philosophical difference between outcome and need is of paramount importance. When looking at software, organisations can often tie themselves in knots when trying to delineate between their outcomes and their needs, and this is something that must be understood to successfully navigate ‘build vs buy’.
If an organisation wants to build an AI solution, then it must understand if it has the capability and expertise to do so. Many have phenomenal software factories that can build exquisite software products and platforms for internal use, but AI software, and specifically some of the challenges that users want to address with it – data analysis, for instance – is not necessarily a software engineering problem, rather it is a data science problem. AI is the technology enabler, but data science is the fundamental ability, the fundamental capability that underpins an AI solution, and that expertise is expensive.
The expertise required is also not widely available, and so without having strong data science capabilities inside an organisation attempting to build an AI solution will likely be an ask or a task too far, and that is without taking into consideration the financial resources required.
Bespoke solutions are expensive to build and support. Aside from the initial build costs, through-life support and maintenance must be considered, as must the resources and investment that will be required to adapt that software as business requirements change. Keeping a solution up to date and relevant can be an expensive and resource draining proposition.
It is also worth considering philosophically that there is an argument to make that ‘Big IT’ as a concept is almost dead outside of cross-enterprise systems, such as customer relationship management and enterprise resource planning software.
Organisations must determine if it is possible to build solutions using no-code and low-code commercial off-the-shelf software, moving away from monolithic engineering with expensive and complex solutions to a ‘building blocks’ concept for delivering capability.
Successfully building a bespoke AI capability in-house is fraught with difficulties, and even with the deepest pockets and extensive expertise, it is likely that an organisation will fail to meet its requirements. Likewise, opting for a purely off-the-shelf solution could lead to compromise if the tech provider selected is not agile and built to deploy rapid innovation.
In the buy v build debate a blended, ‘adaptive enablement’ approach is arguably the most likely to succeed. This approach requires an organisation to ready itself for adopting AI capabilities by assessing its needs and outcomes, evaluating its readiness, and developing an effective action plan, then, by engaging with the AI sector – where the expertise, innovation, and ‘smarts’ lie – they can introduce effective and impactful AI capabilities that meet their specific demands. However, organisations must be prepared to be agile and to iterate, because the world of AI is changing constantly and at a rate like no other.