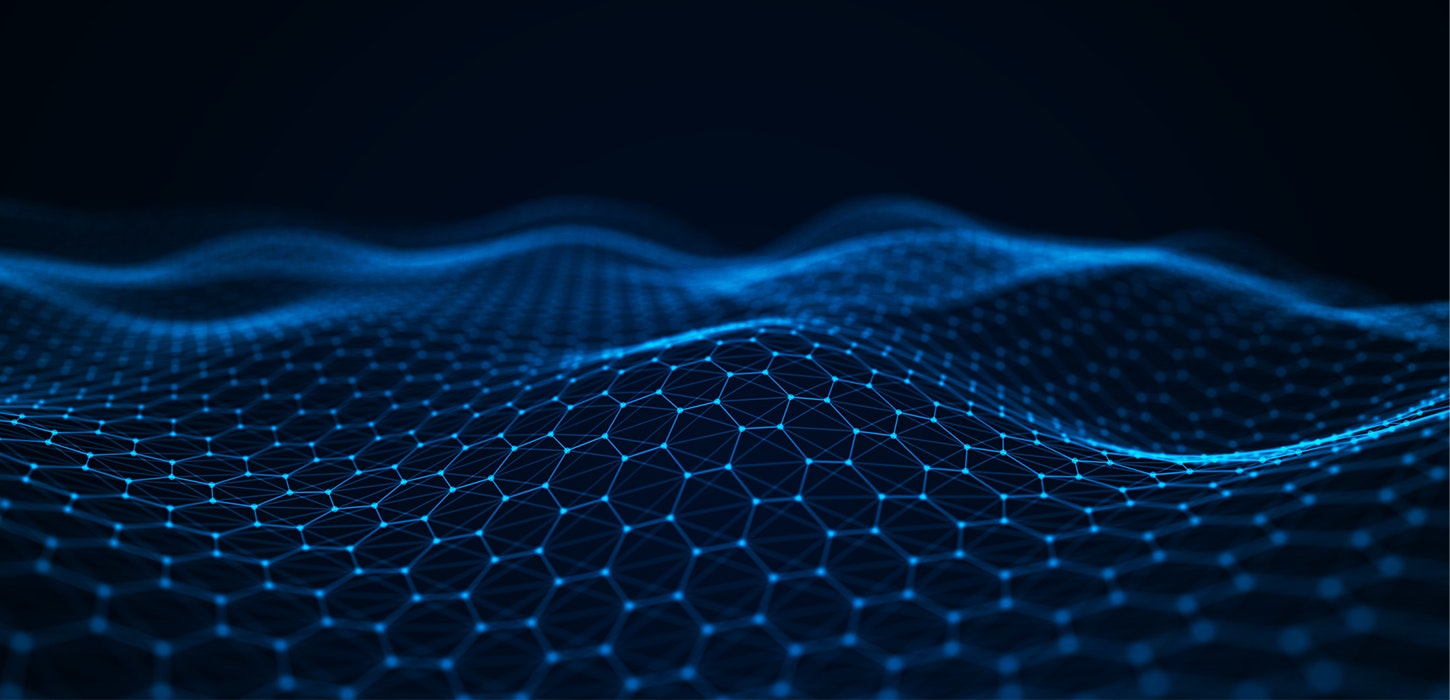
3 focus areas to enhance AI safety in the world of Generative AI
Adarga’s Dr. David Green outlines 3 key areas of focus to ensure that the risks of AI technologies are minimised whilst maximising on the benefits that these new developments bring to society.
While the phrase ‘AI Safety’ often conjures up images of killer robots and other science fiction tropes, these Hollywood depictions are far removed from the technologies that are already impacting our daily lives. Generative AI, which can be used to rapidly create content such as text and images, has now dramatically impacted the way the public interacts with and understands AI. ChatGPT is a prime example of this.
Organisations and consumers around the world are already reaping the benefits of Generative AI. With the right measures in place, these technologies can be leveraged safely and responsibly.
In this post, we identify three key areas that need to be and can be addressed with present-day technology and discuss practical, concrete steps that can be put in place to ensure the recent developments in AI are of maximal benefit to society.
Robust Guardrails
Organisations deploying Generative AI models can and should put in place robust guardrails that help to produce trustworthy outputs and minimise the risk of hallucinations. An example of a hallucination would be a chatbot producing a ‘confidently’ wrong answer to a user.
These guardrails can be put in place to detect when a model has produced a confabulation. While there are many possible approaches to dealing with model hallucination, we will provide one as an example for a ChatGPT-style chatbot. In this example, imagine a user asks a question about history. A model could, after producing a response, extract events from that response and compare those events against a database of known facts (using what is known as Retrieval Augmented Generation or RAG). How well the events in the generated response match the events in the database could be given a score. Generated responses with a low score could be discarded and the user given a warning, or perhaps another attempt could be made automatically to generate a better response. This is just one example, but there are many forms these guardrails could take.
To further minimise the risks related to hallucinations, models that are intended to act as a source of information, again like ChatGPT, could be trained to avoid ‘editorialising’ and instead to focus on the presentation of what can be found in the training data. This could be further enhanced by RAG database methods. The AI could be trained to prefer only generating answers that match what was found in the fact database. This is subtly different from the previous point, as this would involve building the guardrails into the trained model at a more fundamental level. More research is required in this area to ensure that it could be done effectively but also to enhance the power of RAG-based information retrieval.
Explainable AI, data providence and sourced ouputs
Although mis/disinformation exists online, it often is difficult to get the ‘truth’ even with the best sources available. Different viewpoints of the same event may be caused merely by the inherent subjectivity of interpretation. Below we discuss several methods for dealing with this issue, related to both model training and to the presentation of information.
Generative AI carries its own subjectivity and biases reflective of the data used to train the model. To account for this, the data used for training a model should be known and model training should be reproducible. While technology exists to deal with this today, responsible AI organisations need to put in place formal standards for tracking what data was used. This would enable for models trained on poor data sets to be avoided or for datasets found to contain questionable material to be removed from training. A rigorous approach such as this would be akin to best practice in embedded software design where ‘repeatable builds’ are used to ensure that the path from code to an executable program is reproducible. These sorts of approaches enable the origin of software artifacts to be traced. International best-practice standards could be developed and followed or even enforced.
Furthermore, when a model presents information to a user, technology could be developed to make clear what part of the training data was responsible for the outputs of the model. Techniques already exist for understanding how the weights (parameters that form part of the internal representation) of a model are impacted by different parts of a training data set. Expanding on these methods, models could output which parts of the training data were responsible for their outputs.
Accompanying outputs with citations or ‘sourced outputs’(where you can actually see/read and corroborate the original source), in the same way more formal, human-generated media does already, is fundamental. It provides users with a safer and more transparent means of consuming this type of information.
Enhanced harm reduction methodologies
While the previously discussed guardrails could check the veracity of information, guardrails can also be put in place to perform content moderation when it comes to malevolent actors producing illicit images, deep fakes or other anti-social content.
For example, supervised deep neural networks trained to detect illicit images can be run over the outputs from a Generative AI model. This would enable any images that trigger the content filter not to be served to the end user. These technologies already are in use today but could be made more robust with additional investment and research effort.
Watermarking technology could also be developed to ensure that the source model of a particular AI generated output was known. While open-source models could be built that do not inject watermarks into their outputs, a system like the digital certificate system used by websites could be used to guarantee that one was interacting with a model known to be trained and deployed in a responsible way.
Conclusions
Regulation is struggling to keep pace with rapid technological progress. As such, organisations must hold themselves accountable for the safe deployment of AI. Aside from just acting within the bounds of the law, all organisations within a free society have a responsibility to act in an ethical and professional manner. This sort of self-regulation and accountability could be enhanced by government guidelines and international standards covering AI best practice.
The challenge of the government is to ensure that, while harm is minimised, the UK remains a centre for innovation and a pioneer operating at the frontier of all aspects of AI, including safety. This is a substantial challenge that can be addressed by increasing industry engagement and identifying and implementing concrete strategies to address AI Safety.
To learn more about AI safety and responsible AI practices, which sit at the heart of Adarga’s mission as an information intelligence specialist, get in touch via hello@adarga.ai.