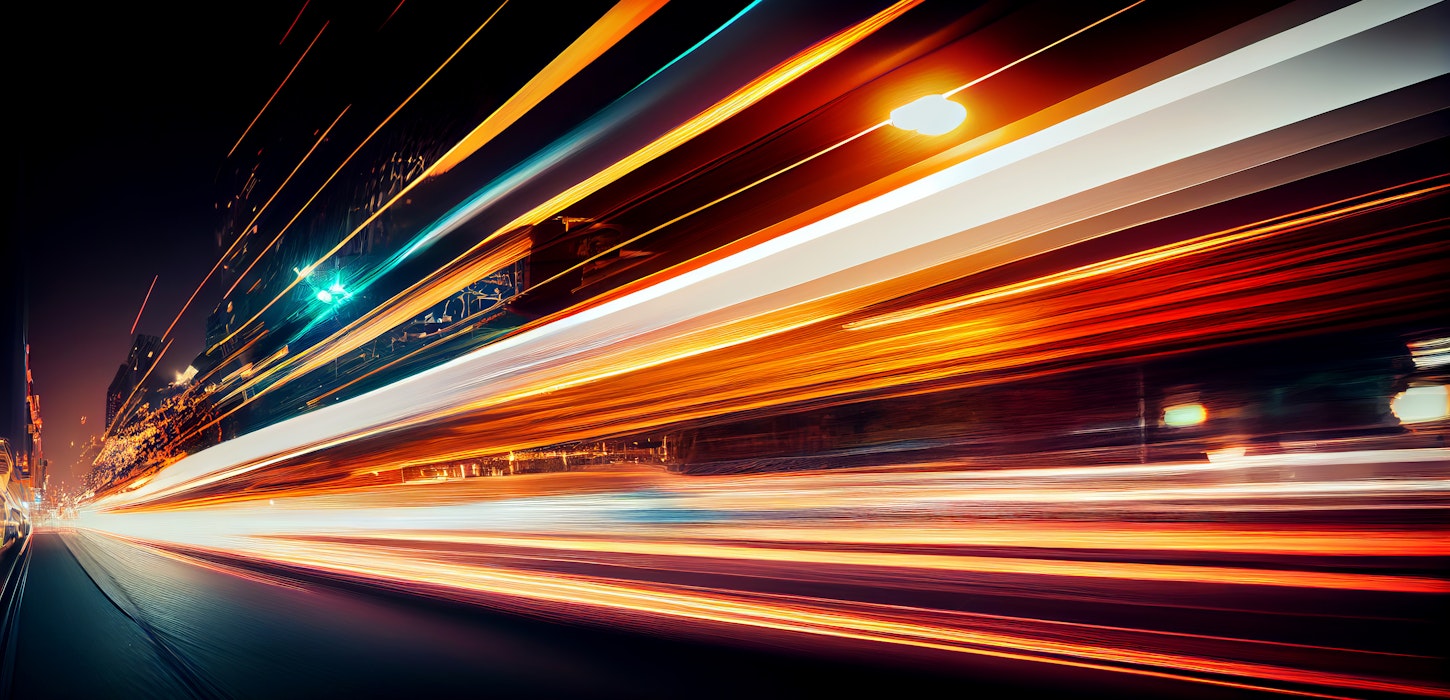
'Don't Blink!': How the UK can take a lead in the Defence AI race
Written by Robert Bassett Cross MC, CEO & Founder of Adarga, and Dr. Keith Dear, @kpd_musing on X.
The UK finds itself at a strategic and technological crossroads and must act boldly to ensure our future security and economic prosperity. We outline our recommendations for how to stay ahead in this vital race and successfully face up to a scale of both opportunity and threat not seen in decades.
Introduction / overview
The UK’s Strategic Defence Review begins this month. Issues compete for attention. Some argue that artificial intelligence (AI) is all hype, undeserving of prioritisation.[1] Others that it’s all too difficult, and since the UK Ministry of Defence (MoD) has proved so consistently poor at buying, developing and scaling emerging technology, with war perhaps imminent and so many other failures to address, the MoD should give-up on technological transformation.[2]
We believe this position is only sustainable if your eyes have been closed to, or your gaze averted from, the rate, direction, and extent of progress in AI over the last 15 years or so. In our experience, even if your eyes are open and your focus constant, the speed is such that you dare not blink for fear that further advances will disorient, destabilise and perhaps defeat your current strategy. We hope the title of this article ‘Don’t Blink’ – can be something of a mantra within the Defence Review Team, in discussing AI development and adoption – and the wider scientific, technological, economic, military, and social disruption it promises and threatens in equal measure. During the writing of this article, OpenAI released its ‘o1’ reasoning model, which the San Francisco-based AI lab claims significantly outperforms its previous flagship model GPT-4o, better able to work its way through complex problems and execute more sophisticated strategies. It is likely to have broken yet more benchmarks by the time you read this. Don’t Blink.
The Defence adoption gap
Targeting-cycles are being sped-up by analytical decision-support systems. Intelligence, surveillance and reconnaissance information is sifted, structured, and shared through AI-analytics. Uncrewed F-16s take to the skies to dogfight. Combined Air Operations involving thousands of drones overwhelm Russian ISR, electronic warfare and air defences in Kursk. Surface drones and subsurface drones send the ships of Russia’s Black Sea Fleet to the bottom or back into harbour. Ukrainian drones conduct battlefield air interdiction, strategic strikes at depth on Russian infrastructure, destroy Russia’s top end air defence systems and force a withdrawal of its most powerful and modern fighter aircraft. All this when few, if any, think military adoption of AI is any more than in its infancy.
To illustrate that adoption gap, consider the following. We already have systems that far exceed human capabilities in specific tasks. Famously, AlphaGoZero (2017) developed superhuman abilities without being trained how to play the game – simply by playing itself – in a game that has more potential moves than there are atoms in the universe and that gets more complicated the longer it goes on. DeepMind’s AlphaStar (2019) conquered the game Starcraft II, requiring the application of game theory, long-term planning, in real time, in vast action space, with partial observability and thus uncertainty. GPT-2 (2019) to GPT-4 (2023) went from pre-schooler to smart secondary school student level in four years. Large Language Models (LLMs) were achieving around 5% accuracy on the Math Benchmark in 2021, by 2022 models were performing at 50%, in 2024 they were achieving >90% accuracy[3] – now we need a new benchmark again. Such performance can be seen in ever more fields of human cognition. Do we really imagine none of this will be relevant to military cognitive tasks? Even if progress in AI stopped tomorrow, and Artificial General Intelligence (AGI) remained out of reach, at its current level, adapted for defence, military AI applied at current levels, at scale, would be completely transformative.
This performance is not just in games and tests. DeepMind’s AlphaFold (2021) system solved the fifty-year old grand challenge of protein folding and is now being used by researchers globally to discover new drugs to solve health challenges, enzymes to tackle plastic pollution, and much else besides. In 2023 materials science was revolutionised when DeepMind’s GNoMe (Graph Networks for Materials Explorations) discovered 2.2 million new forms of crystal, 380,000 years’ worth of knowledge.[4] The company’s AlphaProof (2024) model recently achieved the same level as a silver medallist at this year’s International Mathematical Olympiad (IMO),[5] a feat that involves a model not only able to solve mathematical problems, but also a level of creativity – not the first time a DeepMind model has shown creativity, after AlphaGo’s move 37. AlphaProof and AlphaGeometry (2024), its near gold-medal IMO-standard sister project, also showed AI’s increasing ability to reason.[6] Moreover, AI can now devise novel hypotheses, run experiments, and write up the results in multiple scientific fields, often (not always) as well as, or better than humans.[7]
There is nothing as advanced as these models currently being fielded in UK defence. Military adoption has been significantly slower than the rate of progress. This is partly because of deep scepticism. In 2018 you could still be laughed at in Defence for advocating for AI – it received the same reaction that discussing AGI in defence gets today – dismissed as a juvenile science-fiction fantasy. Perhaps this should not be surprising. It wasn’t just the MoD that was sceptical. GPT-3 (2020) wrong-footed many of the leading experts in the UK, who were talking down LLMs right up to the point it was released.[8] It wasn’t that such progress wasn’t predictable. Many of the leading experts did forecast the rate and direction of progress.[9] Just not those in Government, nor those who Government was willing to listen to.
Other organisations, notably in the private sector, have been much clearer sighted as to the emerging significance of AI. In 2018 Jamie Dimon, CEO and Chairman of J.P. Morgan, made his first explicit reference to AI in his annual letter to shareholders heralding “this is just the beginning”,[10] less than a year after the landmark research paper published by eight Google scientists, ‘Attention Is All You Need’, introduced the transformer approach and now the main architecture of LLMs like GPT. Jamie Dimon has continued to use his annual letter to broadcast his bank’s adoption of AI. J.P. Morgan now has over 400 AI use cases in production in areas such as marketing, fraud and risk and AI is increasingly driving quantifiable value across its businesses and functions. “While we do not know the full effect or the precise rate at which AI will change our business – or how it will affect society at large — we are completely convinced the consequences will be extraordinary and possibly as transformational as some of the major technological inventions of the past several hundred years”.[11] The time where self-harming, unqualified scepticism can be tolerated in Defence must now come to an end.
The rate and direction of progress
There are many more AI achievements, once thought unique to human intelligence, that could be trumpeted. But perhaps better to look beneath the surface, to really see the rate and direction of progress. As investor and economist Leopold Aschenbrenner writes – believing in the transformative effects of AI ‘…doesn’t require believing in sci-fi; it just requires believing in straight lines on a graph.’[12] Some examples:
- Compute growth in recent years has been running at a factor of 300 – 400% per year,[13] it increased 300,000x from 2012-2018.[14] As then OpenAI CTO Greg Brockman put it in Congressional Testimony “…to put that in perspective, that’s like if your phone battery, which today lasts for a day, started to last for 800 years and then, five years later, started to last for 100 million years.”[15]
- The level of compute needed to achieve a given level of performance in LLMs has halved roughly every eight months, or 4000x (390,000%) in the last 8 years due to algorithmic efficiency progress. This outpaces algorithmic progress in many other fields of computing and the 2-year doubling time of Moore’s Law that characterises improvements in computing hardware.[16]
- Today’s supercomputers now exceed the raw computational capacity to simulate the equivalent processing power of the human brain and whole brain emulation will be entirely feasible by 2030.[17]
The rapid growth of compute being applied to develop AI models, coupled with algorithmic progress to make that compute more efficient, alongside ongoing improvements in hardware, suggest we will continue to see rapid progress in AI capabilities.
Much of this expected progress has been mapped out by the Center for a New American Security in its report Future-proofing Frontier AI Regulation.[18] It found:
- Within five years at current trends, the cost to train a model at any given level of capability decreases roughly by a factor of 1,000, or to around 0.1 percent of the original cost, making training vastly cheaper and increasing accessibility.
- By the late 2020s or early 2030s, the amount of compute used to train frontier AI models could be approximately 1,000 times that used to train GPT-4. Accounting for algorithmic progress, the amount of effective compute could be approximately one million times that used to train GPT-4.
Computer Scientist Rich Sutton’s essay The Bitter Lesson reminds us that major breakthroughs in AI have come principally from applying more compute to simple, scalable algorithms, rather than through the development of complex symbolic systems.[19] We should expect further progress, in line with that rate and direction of progress in applying more computation, more efficiently. And all this is without recent innovations such as the use of ‘inference time compute’, which suggests that LLMs can significantly improve their performance without needing larger and larger models or extended pre-training.[20] Similarly, the use of synthetic data is still in its infancy and may help organisations struggling to get their data in order to more rapidly develop and adopt AI-solutions.[21]
Given all this, what is the ‘it’s all hype’ or ‘it’s too difficult’ argument?
To argue that leadership in artificial intelligence doesn’t matter, that investment in its development and adoption should not be the first priority in the Defence Review, one must hold, consciously or otherwise, one of the following four assumptions:
- Humans will continue to outperform machines in all, or the vast majority, of cognitive domains to 2040, or 2050 (the two furthest time horizons to be considered by the Strategic Defence Review, based on the ‘Call for Evidence’). Cognitive domains include, in this sense: strategic planning, preparation, analysis and decisions; the development of operational plans; tactical planning, coordination and the ground, sea, subsurface, air, space and cyberspace-based execution of those plans.
- That humans will continue to outperform ‘Centaur’ teams, humans with significant AI-assistance, across the same cognitive domains, to 2040 or 2050.
- That humans might be outperformed by machines, or centaur human-machine teams, across the strategic, operational and tactical domains within the Review’s timeframe but this does not matter: UK Defence need not prioritise investment in AI development and adoption, it can rely on allies, the private sector, other bits of the public sector, copying enemies’ technology, or surge investment in the future, and catch-up later.
- That allies and adversaries won’t prioritise the development and adoption of AI in defence either, so we can afford to risk not prioritising UK leadership in the field.
For these premises to be true, progress in AI to date would have to slow rapidly and significantly. It would have to be self-evident that AI at the level currently developed has few military applications, or at least none which would provide a significant advantage to the side that applied them effectively. It would have to be sufficiently likely that investment in adoption and/or imitation later would bring at least parity with adversaries, rather than leaving the UK unacceptably less able to secure its interests through the use or threat of use, of military force.
How should the Defence Review consider this? The MoD’s best practice for forecasting the future requires the attribution of probability assessments to uncertain future outcomes.[22] The best way of deriving these forecasts is from crowd judgements and those of ‘superforecasters’[23].
Today, these forecasts estimate the arrival of weak AGI in 2027 (at the 75th percentile of human capabilities),[24] Oracle AGI – one that outperforms us in all cognitive tasks – 21 months later, before the end of 2029.[25] Artificial Super Intelligence (ASI), one that surpasses us in all tasks including those requiring dexterity, locomotion and proprioception, is forecast to arrive within 4.3 years of the weak AGI breakthrough, in 2032.[26] The forecasts for the arrival of all three are trending closer to the present day, not receding into the distant future. Crowd-judgements have been shown to usually outperform those of experts. But AI-expert forecasts also estimate ‘Human Level Machine Intelligence’ (aka Weak AGI) within the Defence Review’s timeline, with the aggregate forecast ascribing a 50% probability of this being achieved by 2040, “down thirteen years from 2060 in the 2022…[survey]”.[27]
It is often said, including by one unnamed senior official speaking under the Chatham House rule at this year’s Chief of the Air Staff’s Air Power Conference, that no-one has defined AGI. But as the forecasts above make clear, this is not true. Definitions are contested certainly, as with concepts from terrorism to art. The contestation of a concept does not invalidate its import or prevent it being discussed. It just requires the concept to be defined contextually, when we speak of it, so we know what the other person means by it, even if we would define it differently. It would be beneficial, indeed it will be essential if the MoD is to assess the prioritisation and risk of AI in the review fully and logically, if it were to define what it thinks AGI and ASI would be.
Furthermore, to understand whether the MoD’s planned and current response – its level of prioritisation and investment in AI – is proportionate to the threat/opportunity, the Review will need to understand the probabilities the MoD is assigning to profound breakthroughs, howsoever defined, and/or what probability threshold or other criteria would have to be true to change the speed and scale of the response. This should not apply only to forecasts of AGI and ASI. It should also consider:
- When and with what probability fleets of ships, submarines, aircraft, spacecraft and vehicles will be fully uncrewed;
- When and with what probability humans will be replaced in respectively, strategic, operational and tactical analysis, planning, command, control and coordination.
Failure to do so will allow the current fudge to continue. Unstated assumptions, implicit in the gap between stated and revealed preferences, continue to hold back progress. Loud talk about the profound importance of AI contrasts with minimal investment. For example:
- High profile but (proportionate to the overall MoD budget) low value investment in R&D – 5% of the Defence Budget, compared to 20% spent on R&D in the most effective software-driven enterprises.[28]
- A drone strategy loudly announced but backed to 2030 with just 0.5% of the defence budget.
- Widely touted experimentation with AI tools, but no widespread adoption and deployment of them.
An approach that we think is indefensible, based on closed eyes and muddled thinking rather than clear focus.
Perhaps the MoD believes, contra to what it says, that AI has a 0% chance of replacing humans, in the functions described, or of reaching AGI or ASI by 2040 or 2050. If it is anything above zero, we should be investing and adapting proportionately to the probabilities of the risk and opportunity such development presents.
A ‘0% chance’ position would likely be justified by pointing to high-profile AI-sceptics. But they are firmly in the minority. The eminent Gary Marcus is perhaps the highest profile. One of the reasons he attracts so much attention is because he is an outlier. The 33,000+ signatories to the Future of Life Institute’s letter calling for a pause in frontier AI development due to the potentially existential risk of AGI or ASI, first published in May 2023, contains a majority of the world’s leading scientists working on AI.[29] Similar calls, letters and articles from large groups of respected scientists in the field continue.[30] The Review might reasonably align with the smaller number of high profile AI/ML sceptics. But they should be clear that they have done so – it’s a risky bet against the consensus among most experts, the pattern of adoption being witnessed in the private sector and those straight lines on graphs.
What we expect to see
On the trends, it seems inevitable that over the coming years we will see (at the very least) much more powerful auto-regressive LLMs, which are able to be employed in specific ways to augment the work of humans, boosting our effectiveness and productivity, and potentially helping us to find novel solutions to challenges.
We are also likely to see smaller, specialised models (as powerful in certain fields as generalised systems now), which are critical to global leadership in war, where size, weight and power are critical considerations, whether in weapons systems and vehicles or deployed HQs and command-and-control centres. Furthermore, as AlphaFold, AlphaGeometry and AlphaProof presage, and OpenAI’s ‘strawberry’ or ‘Qstar’ are said to embody, we may see a shift to neurosymbolic models, where neural networks are shaped, constrained and manipulated by more structured symbolic systems. This too will likely accelerate military adoption. Parameterising and controlling AI’s errors and reducing the opacity of ‘black box’ decision-making in current systems, will increase accountability and reliability, thus reducing the risk of AI-systems operating in unanticipated ways.
One of the milestones we expect to be passed during this period is when AI reaches a point where it can significantly speed up progress in AI development in collaboration with researchers, and where it is able to autonomously and recursively self-improve. From these points onwards, dependent on constraints around compute, power and data – constraints we may be able to overcome – the speed of progress is likely to increase consistently, as better systems contribute to ever-quicker improvement. It was the British mathematician, I.J. Good, a colleague of Alan Turing, who submitted that such a positive feedback loop of self-improvement, with each successive cycle creating a new and more intelligent generation, and each appearing more and more rapidly, would lead to an “intelligence explosion”. One of us recently described this as ‘innovation escape velocity’ – get ahead, and you may never be caught. Fall behind, and you may never catch-up.[31] We believe defence should be planning for such an explosion now, and with far higher priority than any other.
What is most important for policymakers to understand is that this is no longer science fiction, and that progress is likely to be non-linear. Muddling through will not be enough. Blink, miss the moment, and you may never catch up.
The urgency of action
The pace of development, coupled with what is at stake, makes it very clear that policymakers need to come up with solutions now.
A relatively minor technological lead can very quickly become a major – potentially unassailable – technological lead. So whichever country, or countries, have this lead will be the most advantageously placed for the coming decades. We are likely to see a future of AI haves and have-nots.
China has been pursuing ‘intelligentised warfare’ since at least 2017.[32] In 2018, Xi Jingping said “[We must] ensure that our country marches in the front ranks where it comes to theoretical research in this important area of AI and occupies the high ground in critical and AI core technologies.” This is not just announcements. Congressional Testimony in the US records that “China is spending between 1% and 1.5% of its military budget on AI, while the US is spending between 0.1% and 0.2%. Adjusted for the total military budget, China is spending ten times more than the US.”[33]
In contrast the UK MoD devotes somewhere between 1-3% of its military budget to all digital solutions, and we have no way of knowing how much is being spent either on AI R&D or AI development. The Defence Review should rectify this, publishing both relative analyses of our AI spend in comparison to allies and developing public metrics to allow Parliament to scrutinise and understand rates of AI investment and adoption. Without this, and the ensuing public pressure on the Ministry, and career pressure on its senior leaders that will come from public criticism if things don’t improve, incentives will remain as they are, with rhetoric and reality diverging ever more dangerously.
Policymakers and technologists, therefore, should not just be focusing on the end game. Understanding the risks of AGI is essential, taking it seriously likewise. But fixation on AGI will not deliver the security we need against pressing threats from Russia, China and their authoritarian allies. Ceding the technology advantage in AI to a rival nation during the course of the next two decades will have disastrous consequences, allowing the victor to exert a strangle hold over its distribution, control information flows and reap the spoils of economic dominance and influence.
It is perhaps easier to first imagine what losing in this competition looks like for the UK and our allies – loss of control of our markets, trade, vital materials, international law and at the extreme a fundamental shift in the ideological relationship between society and government and control over the dominant operating system for the world. In this fast-emerging ‘Intelligence Age’[34], information – and therefore decision – advantage is crucial. Adoption and iterative development must accelerate now.
How can we win?
The first point is to prioritise this issue in line with its importance. As we have said, this will be the defining dynamic of the coming decades. Therefore, the new Government must ensure that it is a priority issue, from the Prime Minister down. AI will be transformational for all industries and wider society, as well as our defence and national security, and so we must address it through a cross-government approach, in coordination with industry, civil society, and our national security infrastructure.
This is not happening currently. Our systems, policies and processes are not sufficient to be successful in our data- and information-driven world. Our incentive structures are similarly outdated and too often they serve to inhibit, rather than promote, experimentation, innovation and adoption.
Reversing this will not only ensure we have leading military capabilities employing new technologies. The economic and social impacts of prioritising AI development in the UK will be game changing. The technology will revolutionise our ability to deliver public services, allowing us to achieve far more with fewer resources, and far more effectively. It will boost productivity by working alongside humans, multiplying individual workers’ effectiveness. It will be the most in-demand technology of the coming decades, offering those countries that lead development an unprecedented growth and export opportunity.
Defence cannot do this alone. Maintaining a pro-innovation regulatory landscape will need a wider Government effort. Labour promised to put the work of the AI Safety Institute on a statutory footing and the Party has suggested it will introduce AI-specific legislation in the future. The UK currently has a strong global reputation for its pro-innovation approach to AI, balancing this with efforts to identify and introduce guardrails on the most advanced systems. The benefits of this reputation should not be underestimated, particularly as it relates to attracting global investment. Any implementation of regulation or legislation should be carefully considered so as not to undermine our existing advantage. Post-Brexit, and building on the UK’s position as a leading legal and financial centre, we have an opportunity to set a global precedent and export our standards and laws.
We must encourage research and innovation at all levels of the AI ecosystem. The UK’s AI ecosystem is made up of a broad range of businesses, research institutions and academia. The industry segment of this consists of a mix of some of the largest labs in the world, scale-ups, innovative start-ups and inventive builders. As the rapid growth of AI start-ups like OpenAI, Mistral and others in recent years, alongside breakthroughs at leading labs like Google DeepMind has shown, significant advances can come from all parts of this ecosystem. But this needs more focus on applied research solving real problems or challenges in an interdisciplinary manner. Our universities have the potential to be the birthplace of world-leading advances and spin-out companies, but too many reports on how to do this lie unimplemented. For example, the Government’s Independent Review of University Spin-out Companies made recommendations on the percentage of equity UK universities should take in spin-outs, recommending around10% for the typical deal.[35] But little seems to have changed with the University of Oxford, for example, continuing to take on average 24% equity stakes in its spin-outs.[36] Similarly, much could be learned from existing studies on improving innovation effectiveness, such as NASA’s Venture Capital Study,[37] noting NASA’s success in harnessing the private sector to help fund US space programmes in recent years.
Nurturing might go further to ensure that we realise the full potential of the ecosystem. In the case of France’s Mistral, it is worth noting that its success has been influenced not just by the brilliance of its founders, but also through the dedicated support of the Macron government, which has made it a national champion, used its success to boost Paris’ reputation as an AI hub, in-turn attracting new private investment, and protected its ability to operate and innovate during regulatory development at EU level. Labour’s commitment to Industrial Strategy bodes well, here.
Industrial strategy will also need to reduce the UK’s exposure to supply chain vulnerabilities. Recent years have exposed the fragility of global supply chains, and Chinese threats to Taiwan (coupled with increasing protectionism of IP and skills) imperil our ability to access the hardware needed for continued AI development. This will need industrial policy to look at the UK’s dependencies and understand which are critical and need to be onshored, or friend-shored, with contracts in place for assured access in time of crisis.
Unless the UK can remain at the forefront of AI development, our access to leading technologies is not guaranteed. It is imperative, therefore, that we create national champions – akin to Mistral in France – which can develop leading IP, continue to grow, and provide the strategic advantage needed in this global race.
If we are to succeed in this AI-race, the academic sector itself will need research organisations designed and rewarded for excellence. Former Downing Street advisor James Phillips’ ‘Lovelace Institutes’ applied the lessons of the global metascience movement to improve our universities’ performance in key technology sectors[38], is another example of a proposal ready to be implemented. It just needs will and prioritisation.
It also needs honesty. As Phillips and Professor Paul Nightingale have described, UK university performance is often far worse than superficial aggregated national science metrics suggest. In AI for example, “…without DeepMind the UK’s share of the citations amongst the top 100 recent AI papers drops from 7.84% to just 1.86%...[and]… In every case examined, a single institution such as MIT or DeepMind could be found that matched the entire UK academic performance.” [39] Labour’s willingness to drop boosterism and be far more clear-eyed on the reality of the UK’s challenges could be a key strength in the Review, if it can be as clear-eyed, hardnosed, and unflinching in controversial areas such as these as it has been on public finances.
Collaborating with our global allies will be crucial too. While the UK should ensure that it has its own home-grown successful AI ecosystem, the nature and scale of the race to national AI power means that we are competing against adversaries, including China, whose AI investment will continue to outpace our own. Because of this, and because of the risks of losing this race, the UK should collaborate closely with our allies, including through existing multinational alliance structures such as (J)AUKUS, Five Eyes and NATO, to create a unified global AI alliance. Doing this will mean combining the financial and research power of our nations and give us the greatest chance of success.
Conclusion
The speed and progress we are witnessing in AI will mean the country, or countries, who lead AI development will reap the spoils of economic dominance and influence and control access to the world’s most powerful technology. In this race, advantage is key, even if it is marginal or fleeting. Even a slight technological lead will translate into supremacy and real-world impact. This makes this the most important factor in military and economic advantage in the coming decades. To date, the UK has not faced up to the reality of this situation.
The next 20 years are therefore critical. We are involved in and threatened by converging geopolitical conflicts and competition. We are arming Ukraine in a fight against one of our primary adversaries on the border of NATO. Another of our adversaries, China, is menacing an island which is the world’s semiconductor factory – and therefore the enabler of AI development. In the Middle East, Iranian proxies are targeting UK, US and allied forces, as well as disrupting global supply chains by targeting merchant shipping. Combined, these represent a scale of threat unknown for decades. Those decades have created a malaise: a presumption that conflict between significant powers, on a global scale, is a thing of the past. Recent years have been an antidote to that malaise, but we still display a lack of urgency in our preparations for an information age conflict.
As Ukraine has shown, in adversity there is no alternative but to innovate to survive. The UK has the opportunity to kickstart this intensity of innovation before the moment of no return – ‘out of contact’ before the shooting starts. Whilst Ukrainian armed forces have been incredibly agile, continually developing technology and tactics since the onset of the Russian invasion in February 2022, deeper national innovation transformation started in 2014 and their defining experience the lessons drawn from the 2014-2022 Donbas War, whilst also not overlooking the vital importance of the cultural, organisational and doctrinal adaptation processes that started as early as the 1990s.
Given that marginal advantage can be devastating, it has to be secured, both now and continuously. This will require a concerted effort to realise the opportunities inherent in the UK’s incredibly strong foundations in AI, which can only be achieved through the mobilisation of the full spectrum of our vibrant AI ecosystem, supported by the financial, regulatory and diplomatic might of the state.
We must approach this issue as we would any other race critical to our national security: with the utmost urgency. Don’t Blink.
A new approach is needed to achieve this. Summarising our recommendations:
1. Make AI Leadership through Adoption the First Priority of the Defence Review:
a. Recognise AI as the defining dynamic of the coming decades and prioritize accordingly.
2. Define AGI and ASI in the Military Context:
a. Establish clear definitions of Artificial General Intelligence (AGI) and Artificial Superintelligence (ASI) relevant to defence applications.
3. Publish the MoD's Assessed Probabilities and Timelines for AI Development:
a. Provide transparency on the MoD’s forecasts, including:
(1) AGI.
(2) ASI.
(3) Fully autonomous command-and-control systems.
(4) Autonomous R&D systems.
(5) Fully autonomous air, sea (surface and subsurface), and land systems matching or exceeding crewed systems' performance.
4. Publish Metrics for Tracking AI Progress:
a. Develop and release metrics to assess progress in AI development and adoption, ensuring accountability and enabling Parliamentary scrutiny.
5. Make the UK the Most Attractive Global Location for Dual-Use and Military AI Investment:
a. Maintain and Strengthen Incentives for Military and Security R&D and AI Investment:
(1) Enhance tax incentives, grants, and funding for AI research and development.
b. Provide Stronger, Clearer Demand Signals:
(1) Government to act as an early adopter of AI solutions, signalling commitment to AI integration. It must state how it will scale adoption, what it will spend, to incentivise investors and corporate investment boards to fund military AI solutions.
c. Strengthen the National Security Investment Act:
(1) Enhance protections for critical industries and technologies from malign foreign and adversarial investment risks.
d. Build Lovelace Institutes for AI Leadership in University Research:
(1) Establish dedicated AI research centres to foster innovation and bridge academia with industry.
e. Reduce Exposure to Supply Chain Vulnerabilities:
(1) Onshore or friend-shore critical dependencies and secure contracts for assured access during crises.
(2) Provide analytical support for supply chain tracking.
(3) Undertake an assessment of the UK’s critical vulnerabilities, and where others are dependent on UK suppliers.
6. Encourage Research and Innovation Across the AI Ecosystem:
a. Implement recommendations from the Government’s Independent Review of University Spin-out Companies.
b. Apply lessons from studies like NASA’s Venture Capital Study to improve innovation effectiveness. Dust off existing reports and recommendations and ensure implementation.
c. Nurture start-ups and scale-ups to become UK national champions, similar to France's support for Mistral.
7. Adopt a Cross-Government Approach to AI:
a. Provide coordination between industry, civil society, and national security sectors to address AI's societal impacts, with MoD acting as convening authority for AI in Defence and National Security.
8. Collaborate with Global Allies:
a. Work through alliances like (J)AUKUS, Five Eyes, and NATO to combine financial and research capabilities at a scale commensurate with the opportunity and risk.
9. Develop an Industrial Strategy Focused on AI:
a. Adoption across existing defence enterprise (Government, MoD, armed forces, industry).
b. Invention across the UK defence enterprise.
c. Address dependencies on critical hardware and software under various geopolitical scenarios.
10. Update Incentive Structures Within the MoD:
a. Reform outdated policies that inhibit experimentation, innovation, and adoption of new technologies, with explicit consideration of the incentives acting at individual level – social, career, financial, organisational, both internally and externally.
11. Plan for Non-Linear Progress in AI:
a. Being ‘AI ready’ does mean ensuring data is structured, cleaned and transformed but also requires:
(1) Education in the speed of advance in AI capabilities, similar to other routine threat and intelligence briefings.
(2) Getting beyond muddling through, to clear programmes that aim to be first in those areas the MoD judges will be most critical to national security and defence. This will require the level of ambition we showed in funding for HS2 or the Global Combat Air Programme (GCAP), not that allocated to IT programmes.
References
1 Lindsay, J.R., 2024. War Is from Mars, AI Is from Venus: Rediscovering the Institutional Context of Military Automation (Winter 2023/2024); Hunter, C. and Bowen, B.E., 2024. We’ll never have a model of an AI major-general: Artificial Intelligence, command decisions, and kitsch visions of war. Journal of Strategic Studies, 47(1), pp.116-146; https://sciencebusiness.net/news/militaries-are-still-waiting-ai-revolution Ford, M., 2024. Let's face it, military AI isn't going to work. Datacentres already consume 20 per cent of Ireland's electricity production.... X (formerly Twitter), 14 September. Available at: https://x.com/warmatters/status/1834931079121174581 [Accessed 16 September 2024].
2 Sylvia, N., 2024. Can Technology Solve the UK Military’s Problems? Royal United Services Institute (RUSI), 22 August. Available at: https://www.rusi.org/explore-our-research/publications/commentary/can-technology-solve-uk-militarys-problems [Accessed 16 September 2024].
3 Neyshabur, B., 2024. 2.5 years ago, our team decided to improve reasoning capabilities of LLMs & Hendryks MATH.... X (formerly Twitter), 19 May. Available at: https://x.com/bneyshabur/status/1834931079121174581 [Accessed 16 September 2024].
4 Materials science is a discipline born in the 1940s. In wartime it was applied interdisciplinary research collaboration for the development of new weapons and military systems. The implications of the GNoMe revolution for defence should be clear.
Before GNoMe, human experimentation had produced 20,000 computationally stable crystals in the history of science to that point, while other attempts to apply computational methods had still only yielded a further 48,000. Deepmind has open sourced the results.
5 AlphaProof and AlphaGeometry teams, 2024. AI Solves IMO Problems at Silver Medal Level. DeepMind Blog, 25 July. Available at: https://deepmind.google/discover/blog/ai-solves-imo-problems-at-silver-medal-level/ [Accessed 1 September 2024].
6 AlphaProof and AlphaGeometry teams, 2024. AI Solves IMO Problems at Silver Medal Level. DeepMind Blog, 25 July. Available at: https://deepmind.google/discover/blog/ai-solves-imo-problems-at-silver-medal-level/ [Accessed 1 September 2024].
7 Mollick, E., 2024. Four Singularities for Research. One Useful Thing blog, 26 May 2024. Available at: https://www.oneusefulthing.org/p/four-singularities-for-research [Accessed 18 September 2024].
8 Blair, T. and Hague, W., 2023. A New National Purpose: AI Promises a World-Leading Future of Britain. Contributors: Furlong, P., Garson, M., Innes, K., Iosad, A., Large, O., Levin, J.C., and Zandermann, K. Tony Blair Institute for Global Change. Available at: https://institute.global/insights/politics-and-governance/new-national-purpose-ai-promises-world-leading-future-of-britain [Accessed 1 September 2024].
9;AI Impacts, 2015. AI Timeline Surveys. Available at: https://aiimpacts.org/ai-timeline-surveys/ [Accessed 16 September 2024]. or (e.g.) Ford, M., 2018. Architects of Intelligence: The truth about AI from the people building it. Packt Publishing Ltd.
10 Dimon, J, 05 April 2018. Chairman and CEO Letter to Shareholders 2017, J.P. Morgan.
11 Dimon, J, 08 April 2024. Chairman and CEO Letter to Shareholders & Annual Report 2023, J.P. Morgan.
12 Aschenbrenner, L., 2024. From GPT-4 to AGI. Situational Awareness.AI. Available at: https://situational-awareness.ai/from-gpt-4-to-agi/ [Accessed 1 September 2024].
13 Ho, A., Sevilla, J., and Roldán, E., 2024. Training Compute of Frontier AI Models Grows by 4.5x Per Year. Epoch AI. Available at: https://epochai.org/blog/training-compute-of-frontier-ai-models-grows-by-4-5x-per-year [Accessed 16 September 2024].
14 OpenAI, 2018. AI and Compute. Available at: https://openai.com/index/ai-and-compute/ [Accessed 1 September 2024].
15 U.S. House of Representatives, 2018. Artificial Intelligence: With Great Power Comes Great Responsibility. Joint Hearing before the Subcommittee on Research and Technology & Subcommittee on Energy, Committee on Science, Space, and Technology, House of Representatives, One Hundred Fifteenth Congress, Second Session, June 26, 2018. Serial No. 115-67. Available at: https://www.govinfo.gov/content/pkg/CHRG-115hhrg30877/pdf/CHRG-115hhrg30877.pdf [Accessed 1 September 2024].
16 Ho, A., Besiroglu, T., Erdil, E., Owen, D., Rahman, R., Guo, Z.C., Atkinson, D., Thompson, N. and Sevilla, J., 2024. Algorithmic Progress in Language Models. Epoch AI. Available at: https://epochai.org/blog/algorithmic-progress-in-language-models [Accessed 16 September 2024].
17 See Kurzweil, R, The Singularity is Nearer and Sandberg, A and Bostrom, N, Whole Brain Emulation - A Roadmap, 2008.
18 Center for a New American Security (CNAS), 2023. AI Trends: Defense and Security Implications. Available at: https://s3.us-east-1.amazonaws.com/files.cnas.org/documents/CNAS-Report_AI-Trends_FinalC.pdf [Accessed 16 September 2024].
19 Sutton, R.S., 2019. The Bitter Lesson. Available at : http://www.incompleteideas.net/IncIdeas/BitterLesson.html?ref=blog.heim.xyz [Accessed 1 September 2024].
20 Snell, C., Lee, J., Xu, K. and Kumar, A., 2024. Scaling llm test-time compute optimally can be more effective than scaling model parameters. arXiv preprint arXiv:2408.03314.
21 Liu, R., Wei, J., Liu, F., Si, C., Zhang, Y., Rao, J., Zheng, S., Peng, D., Yang, D., Zhou, D. and Dai, A.M., 2024. Best practices and lessons learned on synthetic data for language models. arXiv preprint arXiv:2404.07503.
22 Development, Concepts and Doctrine Centre, 2023. Joint Doctrine Publication 2-00: Intelligence, Counter-intelligence and Security Support to Joint Operations. 17 August 2023. Available at: https://www.gov.uk/government/publications/jdp-2-00-understanding-and-intelligence-support-to-joint-operations#full-publication-update-history [Accessed 18 September 2024]
23 Tetlock, P.E. and Gardner, D., 2016. Superforecasting: The art and science of prediction. Random House; Mandel, D.R. and Barnes, A., 2018. Geopolitical forecasting skill in strategic intelligence. Journal of Behavioral Decision Making, 31(1), pp.127-137; Friedman, J.A., 2019. War and chance: Assessing uncertainty in international politics. Oxford University Press.
24 Metaculus. When will the first weakly general AI system be devised, tested, and publicly announced? Available at: https://www.metaculus.com/questions/3479/date-weakly-general-ai-is-publicly-known/ [Accessed 27 September 2024].
25 Metaculus, After a weak AGI is created, how many months will it be before the first superintelligent oracle?. Available at: https://www.metaculus.com/questions/4123/time-between-weak-agi-and-oracle-asi/. [Accessed: 27 September 2024].
26 Metaculus. After a (weak) AGI is created, how many months will it be before the first superintelligent AI is created?. Available at: https://www.metaculus.com/questions/9062/time-from-weak-agi-to-superintelligence/ [Accessed 27 September 2024]
27 Grace, K., Sandkühler, J.F., Stewart, H., Thomas, S., Weinstein-Raun, B., and Brauner, J., 2023. 2023 Expert Survey on Progress in AI. AI Impacts Wiki. Available at: https://wiki.aiimpacts.org/ai_timelines/predictions_of_human-level_ai_timelines/ai_timeline_surveys/2023_expert_survey_on_progress_in_ai [Accessed 15 September 2024].
28 Ahlawat, P. et al., How Software Companies Can Get More Bang for Their R&D Buck, 22 November 2019. Available at: https://www.bcg.com/publications/2019/software-companies-using-research-and-development-more [Accessed 18 September 2024]
29 Future of Life Institute, 2023. Pause Giant AI Experiments: An Open Letter. Available at: https://futureoflife.org/open-letter/pause-giant-ai-experiments/ [Accessed 1 September 2024].
30 Eeg. Bengio, Y., Hinton, G., Yao, A., Song, D., Abbeel, P., Darrell, T., Harari, Y.N., Zhang, Y.Q., Xue, L., Shalev-Shwartz, S. and Hadfield, G., 2024. Managing extreme AI risks amid rapid progress. Science, 384(6698), pp.842-845.
31 Dear, K., 2024. The Theory of Winning: Transforming Defence with Technology that Does Not Exist. 26 July 2024, Farnborough International Airshow. Available at: https://www.youtube.com/watch?v=wp6q_HdlDOo
32 Kania, E.B., 2019. Minds at war. Prism, 8(3), pp.82-101.
33 Wang, A., 2023. Statement by Alexandr Wang, Founder and Chief Executive Officer, Scale AI, before the Subcommittee on Cyber, Information Technologies, and Innovation of the House Committee on Armed Services: “Man and Machine: Artificial Intelligence on the Battlefield”. Available at: https://docs.house.gov/meetings/AS/AS35/20230718/116250/HHRG-118-AS35-Wstate-WangA-20230718.pdf [Accessed 16 September 2024].
34 per Altman, Sam, 23 September 2024, The Intelligence Age, personal blog site. https://ia.samaltman.com/
35 Tracey, I. and Williamson, A., 2024. Independent Review of University Spin-out Companies: Final Report and Recommendations. UK Government. Available at: https://assets.publishing.service.gov.uk/media/6549fcb23ff5770013a88131/independent_review_of_university_spin-out_companies.pdf [Accessed 16 September 2024].
36 Smith, T., 2023. How Bad Are Oxford University’s Spinout Policies? Sifted. Available at: https://sifted.eu/articles/oxford-university-spinout-policies [Accessed 16 September 2024].
37 Broadwell, M. and Clements, G., 2019. NASA Space Portal: Advancing Economic Development of Space. NASA. Available at: https://www.nasa.gov/wp-content/uploads/2019/10/space_portal_broadwell_and_clements.pdf [Accessed 16 September 2024].
38 Phillips, J., 2022. My Metascience 2022 Talk on New Scalable ‘Technoscience’ Laboratory Designs, and Potential Upcoming ‘New National Purpose’ Reports. Substack. Available at: https://jameswphillips.substack.com/p/my-metascience-2022-talk-on-new-scalable [Accessed 16 September 2024].
39 Phillips, J.W. and Nightingale, P., 2024. S&T: Is the UK a World Leader? Substack. Available at: https://jameswphillips.substack.com/p/s-and-t-is-the-uk-a-world-leader [Accessed 16 September 2024].